Machine learning and domain adaptation to monitor yoghurt fermentation using ultrasonic measurements
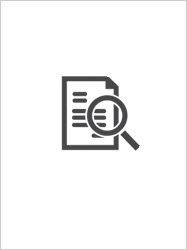
Erişim
info:eu-repo/semantics/openAccessTarih
2023Erişim
info:eu-repo/semantics/openAccessÜst veri
Tüm öğe kaydını gösterKünye
Machine learning and domain adaptation to monitor yoghurt fermentation using ultrasonic measurements Bowler, Alexandera;Ozturk, Sameta, b;di Bari, Vincenzoc;Glover, Zachary J.d;Watson, Nicholas J.Özet
In manufacturing environments, real-time monitoring of yoghurt fermentation is required to maintain an optimal production schedule, ensure product quality, and prevent the growth of pathogenic bacteria. Ultrasonic sensors combined with machine learning models offer the potential for non-invasive process monitoring. However, methods are required to ensure the models are robust to changing ultrasonic measurement distributions as a result of changing process conditions. As it is unknown when these changes in distribution will occur, domain adaptation methods are needed that can be applied to newly acquired data in real-time. In this work, yoghurt fermentation processes are monitored using non-invasive ultrasonic sensors. Furthermore, a transmission based method is compared to an industrially-relevant non-transmission method which does not require the sound wave to travel through the fermenting yoghurt. Three machine learning algorithms were investigated including fully-connected neural networks, fully-connected neural networks with long short-term memory layers, and convolutional neural networks with long short-term memory layers. Three real-time domain adaptation strategies were also evaluated, namely; feature alignment, prediction alignment, and feature removal. The most accurate method (mean squared error of 0.008 to predict pH during fermentation) was non-transmission based and used convolutional neural networks with long short-term memory layers, and a combination of all three domain adaption methods. © 2023 The Authors
Cilt
147Bağlantı
https://www.sciencedirect.com/science/article/pii/S0956713523000221?via%3Dihubhttps://hdl.handle.net/20.500.12440/5821