ReCRNet: a deep residual network for crack detection in historical buildings
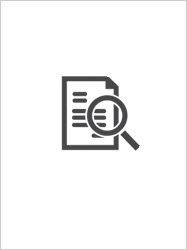
Erişim
info:eu-repo/semantics/closedAccessTarih
2021Erişim
info:eu-repo/semantics/closedAccessÜst veri
Tüm öğe kaydını gösterÖzet
In historical buildings, surface cracks are important indicators of potential structural damage. Natural disasters and indirect human factors, which are frequently encountered in recent periods, negatively affect historical buildings and structures. Fast and cost-effective crack detection methods play a key role in structural health monitoring of historical buildings. This paper presents methodologies for identifying concrete cracks using deep learning. We propose ReCRNet, a deep learning architecture designed for classifying images of cracks. The performance of the proposed method is evaluated and compared with state-of-the-art methods such as AlexNet, VGG 19, linear support vector machine (SVM), and decision tree (DT). The results show that ReCRNet achieves better performance in terms of accuracy, precision, recall, F1-score, and receiver operating characteristic (ROC), in comparison with the other crack classifiers. Accordingly, the proposed approach is recommended for automatic monitoring of historical buildings and building condition assessments.