Potato leaf disease detection with a novel deep learning model based on depthwise separable convolution and transformer networks
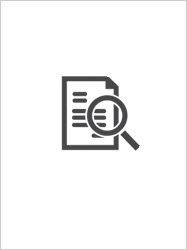
Erişim
info:eu-repo/semantics/openAccessTarih
July 2024Erişim
info:eu-repo/semantics/openAccessÜst veri
Tüm öğe kaydını gösterKünye
Scopus EXPORT DATE: 03 May 2024 @ARTICLE{Catal Reis2024, url = {https://www.scopus.com/inward/record.uri?eid=2-s2.0-85188662434&doi=10.1016%2fj.engappai.2024.108307&partnerID=40&md5=9faa594ee7938f8bc8acd64063b8996f}, affiliations = {Department of Geomatics Engineering, Gumushane University, Gumushane, 29000, Turkey; Department of Computer Engineering, University of Harran, Sanliurfa, 63000, Turkey}, correspondence_address = {H. Catal Reis; Department of Geomatics Engineering, Gumushane University, Gumushane, 29000, Turkey; email: hcatal@gumushane.edu.tr}, publisher = {Elsevier Ltd}, issn = {09521976}, coden = {EAAIE}, language = {English}, abbrev_source_title = {Eng Appl Artif Intell} }Özet
Early diagnosis of plant diseases is essential in reducing economic losses for farmers and increasing production efficiency. Therefore, Computer-Aided Diagnosis (CAD) systems supported by artificial intelligence technologies can be developed to help diagnose diseases quickly and accurately by examining the symptoms and signs in plant leaves. In this study, Multi-head Attention Mechanism Depthwise Separable Convolution Inception Reduction Network (MDSCIRNet) architecture, an image-based deep convolutional neural network, is proposed for classifying potato leaf diseases. The main components of the MDSCIRNet architecture are depthwise separable convolution (DSC) and a multi-head attention mechanism. The proposed architecture has been compared with modern algorithms developed with DSC technology, such as Xception, MobileNet, and deep learning algorithms, such as ResNet101, InceptionV3, and EfficientNetB2, to evaluate its performance in the classification process. In addition, hybrid methods developed with the classical machine learning algorithms Support Vector Machine (SVM), Logistic Regression (LR), Random Forest (RF), Adaptive Boosting (AdaBoost), and MDSCIRNet model, integrated deep learning model, hard voting ensemble learning model. Suggested methods such as these were also used in the experimental process. Moreover, techniques such as Contrast Limited Adaptive Histogram Equalization (CLAHE), Enhanced Super-Resolution Generative Adversarial Networks (ESRGAN), and Hypercolumn were used to improve the image quality of the data set. In the experimental process, the MDSCIRNet deep learning architecture achieved 99.24% accuracy in the study using the original dataset. While a 99.11% accuracy rate was achieved with the integrated deep learning model and hard voting ensemble learning model, the highest success rate of 99.33% was performed in the study conducted with the MDSCIRNet + SVM method. This study contributes to developing new and effective strategies in the agricultural industry for the early diagnosis and control of potato plant diseases. Machine learning-based approaches offer the potential to minimize economic losses and increase productivity in production by allowing farmers to intervene early. © 2024 Elsevier Ltd
Cilt
133Bağlantı
https://www.sciencedirect.com/science/article/pii/S0952197624004652?via%3Dihubhttps://hdl.handle.net/20.500.12440/6214