Pedestrian Inertial Navigation with Multi-Head LSTM Including Attention
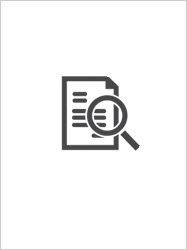
Erişim
info:eu-repo/semantics/closedAccessTarih
2023Erişim
info:eu-repo/semantics/closedAccessÜst veri
Tüm öğe kaydını gösterKünye
M. T. Köroğlu and G. Çetin, "Pedestrian Inertial Navigation with Multi-Head LSTM Including Attention," 2023 Innovations in Intelligent Systems and Applications Conference (ASYU), Sivas, Turkiye, 2023, pp. 1-6, doi: 10.1109/ASYU58738.2023.10296616.Özet
Despite continuous advancements in algorithms and wearable inertial measurement unit (IMU) technology in the last two decades, inertial navigation systems (INS) cannot be used for long-term pedestrian tracking due to the drift issue observed in predictions. Relying on the powerful non-linear regression capabilities of neural networks, Deep Learning (DL) has emerged recently as a potential solution for inertial navigation to cure the curse of the drift. Recent research has demonstrated that DL models trained on public datasets can effectively serve as modern pedestrian INS. The majority of studies utilize torsomounted IMUs and process samples (in transformed coordinates) to indirectly estimate position at lower rates than the IMU sampling rate. In contrast, this study takes a different approach by using raw inertial data and directly targeting the displacement of the pedestrian (including heading change). To accomplish this, a multi-headed structure comprising independent Long Short-Term Memory (LSTM) networks is designed. Additionally, an attention mechanism is incorporated into the network to enhance prediction performance. The proposed model is trained with a dataset collected from a foot-mounted IMU where ground truth data is generated heuristically to provide supervision for the learning process. The results demonstrate that the Multi- Headed LSTM (MHLSTM) model, augmented with an attention mechanism, is capable of generating pedestrian trajectories at the IMU sampling rate, with positioning errors consistently below one meter throughout the duration of the experiment. © 2023 IEEE.