COVID-DSNet: A novel deep convolutional neural network for detection of coronavirus (SARS-CoV-2) cases from CT and Chest X-Ray images
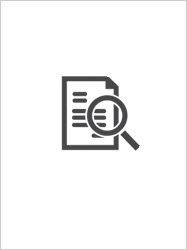
Access
info:eu-repo/semantics/embargoedAccessDate
2022Access
info:eu-repo/semantics/embargoedAccessMetadata
Show full item recordCitation
Reis, H. C., & Turk, V. (2022). COVID-DSNet: A novel deep convolutional neural network for detection of coronavirus (SARS-CoV-2) cases from CT and chest X-ray images. Artificial Intelligence in Medicine, 134 doi:10.1016/j.artmed.2022.102427Abstract
COVID-19 (SARS-CoV-2), which causes acute respiratory syndrome, is a contagious and deadly disease that has devastating effects on society and human life. COVID-19 can cause serious complications, especially in patients with pre-existing chronic health problems such as diabetes, hypertension, lung cancer, weakened immune systems, and the elderly. The most critical step in the fight against COVID-19 is the rapid diagnosis of infected patients. Computed Tomography (CT), chest X-ray (CXR), and RT-PCR diagnostic kits are frequently used to diagnose the disease. However, due to difficulties such as the inadequacy of RT-PCR test kits and false negative (FN) results in the early stages of the disease, the time-consuming examination of medical images obtained from CT and CXR imaging techniques by specialists/doctors, and the increasing workload on specialists, it is challenging to detect COVID-19. Therefore, researchers have suggested searching for new methods in COVID- 19 detection. In analysis studies with CT and CXR radiography images, it was determined that COVID-19-infected patients experienced abnormalities related to COVID-19. The anomalies observed here are the primary motivation for artificial intelligence researchers to develop COVID-19 detection applications with deep convolutional neural networks. Here, convolutional neural network-based deep learning algorithms from artificial intelligence technologies with high discrimination capabilities can be considered as an alternative approach in the disease detection process. This study proposes a deep convolutional neural network, COVID-DSNet, to diagnose typical pneumonia (bacterial, viral) and COVID-19 diseases from CT, CXR, hybrid CT + CXR images. In the multi-classification study with the CT dataset, 97.60 % accuracy and 97.60 % sensitivity values were obtained from the COVID-DSNet model, and 100 %, 96.30 %, and 96.58 % sensitivity values were obtained in the detection of typical, common pneumonia and COVID-19, respectively. The proposed model is an economical, practical deep learning network that data scientists can benefit from and develop. Although it is not a definitive solution in disease diagnosis, it may help experts as it produces successful results in detecting pneumonia and COVID-19.
Volume
134URI
https://reader.elsevier.com/reader/sd/pii/S0933365722001798?token=1418D9307EAE7BD5717B69F8AF6E97058E6B0F2E9BFE81DD709CEB9CC7211EE7488CA955408F61A1DC405779DADE5DC0&originRegion=eu-west-1&originCreation=20230202110132https://hdl.handle.net/20.500.12440/5751