Information theory and machine learning based authentication of flaxseed oil using portable and handheld vibrational spectroscopy sensors
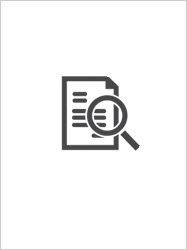
Erişim
info:eu-repo/semantics/openAccessTarih
Chinese JoErişim
info:eu-repo/semantics/openAccessÜst veri
Tüm öğe kaydını gösterÖzet
Flaxseed oil is one of the popular edible oils, and its adulteration has become a concern because of its high commodity value. Therefore, there is a need to detect adulterated flaxseeds with a rapid, non-invasive, and simple method to keep the quality of flaxseed oil. This study aimed to develop a machine learning algorithm and information theory to detect adulterated flaxseed oils by vibrational spectroscopy units combined with chemometrics. Oil spectra were collected with portable/handheld vibrational spectroscopic units and analyzed using conditional entropy, support vector machines (SVM), and soft independent modeling of class analogy (SIMCA) to generate classification models to identify the pureness of oils and partial least square regression (PLSR) to predict the adulterant levels and fatty acid distributions. Conditional entropy showed that two or three wavenumbers/shifts could discriminate the oils using machine learning algorithms with an accuracy of over 95%. SIMCA models determined the oils with an interclass distance (ICD) over 1.1. Specifically, the Fourier transform mid-infrared (FT-MIR) unit provided the best classification of the oils with ICD over 4.9. All instruments showed good performance in predicting fatty acids and adulteration levels with rval≥0.89 and standard error prediction (SEP) ≤1.7%. Overall, these portable/handheld units showed great potential for rapid, simple, and non-invasive monitoring to identify adulterated flaxseed oils. © 2022
Cilt
50Sayı
4Bağlantı
https://www.sciencedirect.com/science/article/pii/S1872204022000196?via%3Dihubhttps://hdl.handle.net/20.500.12440/5638