A Comparative Study of Machine Learning and Deep Learning for Time Series Forecasting: A Case Study of Choosing the Best Prediction Model for Turkey Electricity Production
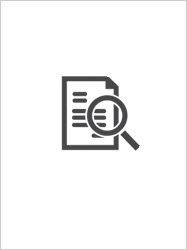
Access
info:eu-repo/semantics/openAccessDate
2019Access
info:eu-repo/semantics/openAccessMetadata
Show full item recordAbstract
Over the last decades, Turkey pays special attention to electricity production to afford its needs. Researchers applied different methodologies including statisticalbased and artificial intelligence-based to correctly predict the future amount of electricity production, consumption, and demand. However,limited researchers focused on Turkey’s electricity production prediction problem as a time series analysis. For this reason, we tackle this problem by considering it as a time series analysis in this study. We have used different methods including traditional machine learning algorithms Support Vector Regression (SVR) and Multilayer Perceptrons (MLP) and a deep learning algorithm Long Short-Term Memory (LSTM) to create a better model for Turkey monthly electricity production dataset. Based on our findings LSTM outperforms SVR and MLP approaches in terms of commonly used statistical error evaluation metrics Son yıllarda Türkiye ihtiyaçlarını kar¸sılayabilmek adına elektrik üretimine yogun bir sekilde dikkat vermektedir. Ara¸stırmacılar elektrik üretim, tüketim ve talep mikarını dogru bir sekilde tahmin etmek için istatistik ve yapay zeka tabanlı yöntemleride içeren birçok farklı metod uygulamıslardır. Sınırlı sayıda ara¸stırmacı Türkiye’nin elektrik üretim tahminleme problemini bir zaman serisi analizi olarak irdelemistir. Bu nedenle bu çalısmada söz konusu problem zaman serileri analizi olarak ele alınmıstır. Bu açıdan çalısmada hem Destek Vektör Makineleri (DVM) ve Çok Katmanlı Nöronlar (ÇKN) gibi klasik makine ögrenimi yöntemleri hem de Uzun Kısa Dönemli Hafıza (UKDH) yöntemi gibi derin ögrenme yöntemi Türkiye’nin üretmesi gereken aylık elektrik üretim miktarını tahmin etmek için kullanılmıstır. Çalısmanın bulgularına dayalı olarak derin ögrenme algoritması istatistiksel hata oranlarına göre diger klasik makine ögrenimi yöntemlerinden daha basarılı sonuçlar vermektedir.
Volume
23Issue
2URI
https://doi.org/10.19113/sdufenbed.494396https://app.trdizin.gov.tr/makale/TXpZNE16WXlNZz09
https://hdl.handle.net/20.500.12440/5251