Symbiotic Organisms Search Algorithm for multilevel thresholding of images
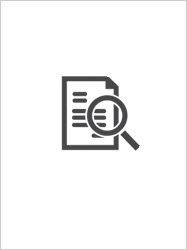
Access
info:eu-repo/semantics/closedAccessDate
2020Access
info:eu-repo/semantics/closedAccessMetadata
Show full item recordAbstract
Thresholding is a frequently used method in image processing because of its consistency and low computational cost. Otsu's and Kapur's methods are two important techniques that were proved to be best thresholding methods. However, they have high computational complexity when extended to multilevel thresholding because of their exhaustively search. Recently, meta-heuristic algorithms have been successfully applied for thresholding problems. In this study, six different meta-heuristic algorithms based on Otsu's and Kapur's functions; Particle Swarm Optimization (PSO), Firefly Algorithm (FA), Symbiotic Organisms Search (SOS), Artifical Bee Colony (ABC), Genetic Algorithm (GA) and grey Wolf Optimizer (GWO) were used for multilevel thresholding problem and compared. Experimental results suggest that SOS, PSO and FA algorithms often have higher fitness values than other algorithms. Especially when more than two threshold values are determined, SOS algorithm mostly gives higher fitness values. PSNR and SSIM results of the algorithms are similar. In terms of computational complexity, the GWO algorithm has the fastest convergence. For standard deviations of objective functions; more stable results were obtained with SOS based on Kapur's function, SOS and PSO based on Otsu's function. Also, SOS based on Kapur's function was found to be the most successful algorithm in the Friedman test. As a result, although the GWO approached faster, the SOS algorithm produced more consistent results for both objective functions. (C) 2020 Elsevier Ltd. All rights reserved.