Giresun ili Aksu Deresi için farklı parametreler kullanılarak yapay sinir ağları ile akış modellemesi ve ileriye dönük akım tahminleri yapılması
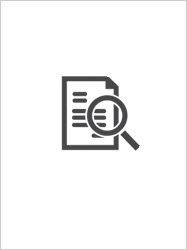
Erişim
info:eu-repo/semantics/openAccessTarih
2015Erişim
info:eu-repo/semantics/openAccessÜst veri
Tüm öğe kaydını gösterÖzet
Bu çalışmada Doğu Karadeniz Havzası içerisinde bulunan Giresun iline bağlı Aksu Deresi için belirlenen 3 farklı Akım Gözlem İstasyonu konumlarında akım modellemesi yapılmıştır. Modelleme için Devlet Su İşleri'nden elde edilen akım verileri, Meteoroloji Genel Müdürlüğü'nden elde edilen yağış ve buharlaşma verileri kullanılmıştır. Oluşturulacak akım modeli için yapay sinir ağları (YSA) metodu tercih edilmiştir. YSA metodu mimarisinde seçilen çok katmanlı yapay sinir ağı (ÇK-YSA) modeli, belirtilen parametrelerin çeşitli kombinasyonlarıyla oluşturulmuş 14 farklı model ile denenmiştir. Denemeler sonucunda tahmin edilen değerler; RMSE, R, RH, E ve OMH hata değerlendirme katsayıları ile değerlendirilmiştir. Her istasyon için en başarılı olan model girdi kombinasyonu çoklu doğrusal regresyon (ÇDR) analizi ile de denenmiştir. Tahmin edilmiş debi değerleri, taşkın koruma tesisleri, hidroelektrik santral tesisleri, arıtma tesisleri gibi yapıların projelendirme aşamasında, en çok kullanılan aşılma olasılıklarına göre belirlenmiş debi değerleri için, gözlem değerleri ile kıyaslanmıştır. Sonuç olarak, ÇK-YSA yönteminin ÇDR yönteminden daha iyi sonuçlar verdiği belirlenmiştir ve gelecek akım verisinin tahmin edilmesinde uygunluğu saptanmıştır. In this study, a flow modelling has been made in three different current observatory stations located the Aksu River Basin in Giresun, a province in Eastern Black Sea Basin. The flow data provided by General Directorate of Hydraulic Works, the precipitation and evaporation data obtained from Turkish State Meteorological Service are used for the modelling. artificial neural network (ANN) method is preferred for the flow modelling that will be created. The multi layered artificial neural network (ML-ANN) method which is selected in the architecture of ANN model has been tested in 14 different models with various combinations of the specified parameters mentioned before. The estimated values from the experiments, are evaluated according to the error coefficients of RMSE, r, RH, E and MAE. The most successful input combinations for each station were tested by Multiple Linear Regression Analysis (MLR) model. During the design phase of structures such as predicted flow results flood protection facilities, hydroelectric plants and water treatment plants, the predicted flow results are compared with the observation values for the most commonly used flow rate which were determined according to the possibility of exceedance. As a result, ML-ANN method was given better results than MLR method and this study shown that ML-ANN method can be used for prediction of future flow datas.
Bağlantı
https://tez.yok.gov.tr/UlusalTezMerkezi/TezGoster?key=OykDDeWBWTL9-Wm52sZBrBXZ0XxzG_bddaSfBtItygE0eU4nmidhYj8C9FHqeYR0https://hdl.handle.net/20.500.12440/2255
Koleksiyonlar
- Tez Koleksiyonu [611]