Advanced Tomato Disease Detection Using the Fusion of Multiple Deep-Learning and Meta-Learning Techniques
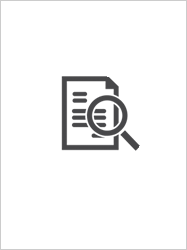
Erişim
info:eu-repo/semantics/openAccessTarih
2024Erişim
info:eu-repo/semantics/openAccessÜst veri
Tüm öğe kaydını gösterKünye
Scopus EXPORT DATE: 05 November 2024 @ARTICLE{Catal Reis2024, url = {https://www.scopus.com/inward/record.uri?eid=2-s2.0-85207243150&doi=10.1007%2fs10343-024-01047-y&partnerID=40&md5=757164f123a7b81f4229cceb73a9a15c}, affiliations = {Department of Geomatics Engineering, Gumushane University, Gumushane, 29000, Turkey}, correspondence_address = {H. Catal Reis; Department of Geomatics Engineering, Gumushane University, Gumushane, 29000, Turkey; email: hatice.catal@yahoo.com.tr}, publisher = {Springer Science and Business Media Deutschland GmbH}, issn = {2948264X}, language = {English}, abbrev_source_title = {J. Crop. Health} }Özet
Automatic and early detection of diseases in crops is important for producers in terms of product yield. Methods are needed to diagnose the disease and select interventions for tomato leaf. This study aims to create a novel framework for automatically extracting tomato leaf disease. The recommended method automatically detects diseases in three classes: healthy, early, and late blight. This study developed a multi-deep and meta-learning fusion framework that automatically combines modern deep-learning algorithms and meta-classifiers to detect tomato leaf disease. The study includes CNN, transformer, and MLP-based models previously trained with the ImageNet dataset and voting classifier (VtC) methods, which are meta-classifiers. Hybrid approaches have been developed using the VtC method and deep learning algorithms such as CoAtNet, FasterNet, FastViT, WaveMLP, and DenseNet201. In addition to the proposed hybrid framework, different types of deep learning models based on CNN, transformer, MLP, transfer learning, and the dataset improved by the CLAHE technique and the original dataset were used to diagnose tomato leaf diseases. While CNN-based modern architectures include DenseNet201, MobileNet, LCNet, GhostNet, CoAtNet, ConvMixer, and FasterNet, transformer architectures include BeiT, FlexiViT, MaxViT, FastViT, and MLP-based architectures include MLP mixer and WaveMLP models. In the classification performed with original images, the proposed method produced incorrect results in only five images among 900 test data. In the classification made with CLAHE images, the proposed hybrid framework produced the most successful result in this study, producing incorrect results in only four images. © Der/die Autor(en), exklusiv lizenziert an Springer-Verlag GmbH Deutschland, ein Teil von Springer Nature 2024.
Bağlantı
https://www.scopus.com/record/display.uri?eid=2-s2.0-85207243150&origin=SingleRecordEmailAlert&dgcid=raven_sc_affil_en_us_email&txGid=2d36f03183fc7352925df05d54ef1872https://hdl.handle.net/20.500.12440/6351