DL-EDOF: Novel Multi-Focus Image Data Set and Deep Learning-Based Approach for More Accurate and Specimen-Free Extended Depth of Focus
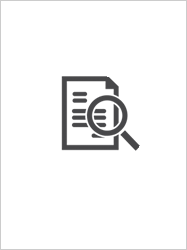
Access
info:eu-repo/semantics/restrictedAccessDate
25.03.2024Access
info:eu-repo/semantics/restrictedAccessMetadata
Show full item recordCitation
Dogan H, Dogan RO, Ay I, Sezen SF. DL-EDOF: Novel Multi-Focus Image Data Set and Deep Learning-Based Approach for More Accurate and Specimen-Free Extended Depth of Focus. J Imaging Inform Med. 2024 Mar 25. doi: 10.1007/s10278-024-01076-z. Epub ahead of print. PMID: 38528289.Abstract
Depth of focus (DOF) is defined as the axial range in which the specimen stage moves without losing focus while the imaging apparatus remains stable. It may not be possible to capture an image that includes the entire specimen in focus due to the narrow DOF in microscopic systems. Extended depth of focus (EDOF) is used to overcome this limitation in microscopic systems. Although the researchers have developed so many EDOF microscope approaches, this research field still has some crucial shortcomings such as high computational costs, complexity and execution time, requiring additional equipment, low precise characterization of curves, and edges in images, varying performance depending on the specimen and microscope, using only gray levels of input images to acquire the pixel’s focus values. In order to minimize these shortcomings and comprehensively analyze the performance of EDOF approaches, a novel multi-focus image data set is generated, and a deep learning-based EDOF microscope approach is proposed in this study. When compared with the state-of-art EDOF approaches, our study provides various crucial contributions such as the first EDOF approach based on unsupervised deep learning, providing more accurate and specimen-free EDOF, generating a novel multi-focus image data, not requiring any pre- or post-processing technique and acquiring the pixel’s focus degrees using deep features. In order to evaluate the effectiveness of the suggested approach, 20 different EDOF approaches are applied to a multi-focus image data set containing 9 image collections (4 synthetic and 5 microscope image collections) in total. Performance analysis metrics with and without requiring a reference image are preferred to identify which EDOF microscope approach can extract more essential details from the multi-focus images for the synthetic and microscope image collections, which are Root Mean Square Error (RMSE), Peak Signal Noise Ratio (PSNR), Universal Quality Index (UQI), Correlation Coefficient (CC), Perception-based Image Quality Evaluator (PIQE), Blind/Referenceless Image Spatial Quality Evaluator (BRISQUE), Extension of Universal Quality Index for N Images (UQIN), and Naturalness Image Quality Evaluator (NIQE). Objective and subjective analysis of this study demonstrates that unsupervised deep learning model is more efficient to transmit crucial details from multi-focus images. Moreover, the suggested EDOF microscope approach with highest PSNR, UQI, CC, UQIN and lowest RMSE, PIQE, BRISQUE, NIQE produces higher performance than the state-of-art approaches.
Collections
- Yazılım Mühendisliği [23]
Related items
Showing items related by title, author, creator and subject.
-
Deep Learning Based Focus Measurement Operator for 3D Imaging in Microscopic Systems
Dogan, Hulya; Dogan, Ramazan Ozgur (IEEE, 2023)Since 3D imaging in microscopic systems makes cell and tissue structures more prominent and visible, it provides higher performance in image processing applications such as automatic segmentation, recognition, classification, ... -
Online learning in vocational school: focus on students' perceptions
Aydin, Sinan; Akkan, Yasar; Arpaz, Ercan; Koparan, Bulent (Elsevier Science Bv, 2015)In this study, we describe vocational school students' practices and perceptions of online learning based on interviews and observations. First, we identify student characteristics within online classes. Then, we report ...