Integrated deep learning and ensemble learning model for deep feature-based wheat disease detection
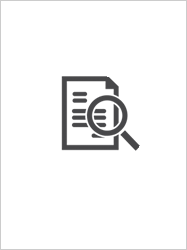
Access
info:eu-repo/semantics/closedAccessDate
2024Access
info:eu-repo/semantics/closedAccessMetadata
Show full item recordCitation
Hatice Catal Reis, Veysel Turk, Integrated deep learning and ensemble learning model for deep feature-based wheat disease detection, Microchemical Journal, Volume 197, 2024, 109790, ISSN 0026-265X, https://doi.org/10.1016/j.microc.2023.109790. (https://www.sciencedirect.com/science/article/pii/S0026265X23014091) Abstract: Early detection of plant diseases is critical to prevent disease spread and assist farmers. Thanks to their high discrimination ability, Convolutional Neural Network (CNN)-based architectures can offer practical solutions in identifying different plant diseases. This study proposes a combined method to classify wheat plant diseases using the Integrated Deep Learning Framework (IDLF) and ensemble learning (EL) model. The proposed method uses pre-trained deep neural networks (ImageNet -based model (w/pre-training)). In addition, in the classification process, the performance of hybrid methods consisting of 13 deep learning architectures (DLA) trained from scratch, pre-trained DLA, deep, and-machine learning models were analyzed. Moreover, the use of hypercolumn, contrast stretching, and “Contrast Limited Adaptive Histogram Equalization (CLAHE)” techniques are used to improve the image quality of the dataset. It is aimed to obtain a strong classification performance by combining these methods. In the experimental process, in the study using the original dataset, the RegNetY080 model trained from scratch had an accuracy value of 97.64%; in the study with the optimized dataset with CLAHE, an accuracy value of 98.33%; in the study performed with the CLAHE-improved dataset and transfer learning method, an accuracy value of 99.58% was obtained. An accuracy of 99.58% was achieved in the proposed hybrid study with the pre-trained RegNetY080 model and Random Forest (RF). The proposed method using IDLF and EL model provided 99.72% accuracy. When the proposed method was applied to the Wheat Leaf Dataset and Wheat Leaf Disease dataset, 97.56% accuracy was obtained for the Wheat Leaf Dataset and 99.43% for Wheat Leaf Disease. The results of this study can be helpful to experts and farmers in the detection of plant diseases. Keywords: Computer vision; Hard voting ensemble learning; Image enhancement techniques; Integrated deep learning frAbstract
Early detection of plant diseases is critical to prevent disease spread and assist farmers. Thanks to their high discrimination ability, Convolutional Neural Network (CNN)-based architectures can offer practical solutions in identifying different plant diseases. This study proposes a combined method to classify wheat plant diseases using the Integrated Deep Learning Framework (IDLF) and ensemble learning (EL) model. The proposed method uses pre-trained deep neural networks (ImageNet -based model (w/pre-training)). In addition, in the classification process, the performance of hybrid methods consisting of 13 deep learning architectures (DLA) trained from scratch, pre-trained DLA, deep, and-machine learning models were analyzed. Moreover, the use of hypercolumn, contrast stretching, and “Contrast Limited Adaptive Histogram Equalization (CLAHE)” techniques are used to improve the image quality of the dataset. It is aimed to obtain a strong classification performance by combining these methods. In the experimental process, in the study using the original dataset, the RegNetY080 model trained from scratch had an accuracy value of 97.64%; in the study with the optimized dataset with CLAHE, an accuracy value of 98.33%; in the study performed with the CLAHE-improved dataset and transfer learning method, an accuracy value of 99.58% was obtained. An accuracy of 99.58% was achieved in the proposed hybrid study with the pre-trained RegNetY080 model and Random Forest (RF). The proposed method using IDLF and EL model provided 99.72% accuracy. When the proposed method was applied to the Wheat Leaf Dataset and Wheat Leaf Disease dataset, 97.56% accuracy was obtained for the Wheat Leaf Dataset and 99.43% for Wheat Leaf Disease. The results of this study can be helpful to experts and farmers in the detection of plant diseases. © 2023 Elsevier B.V.
Volume
194URI
https://www.sciencedirect.com/science/article/pii/S0026265X23014091?via%3Dihubhttps://hdl.handle.net/20.500.12440/6119
Collections
Related items
Showing items related by title, author, creator and subject.
-
Advanced brain tumor analysis: a novel strategy for segmentation and classification using modern computational methods
Reis, Hatice Catal; Turk, Veysel (Springer Science and Business Media Deutschland GmbH, February 2)Despite their low incidence, brain tumors are one of the most invasive cancer types, constituting a significant burden of death and disease in all age groups. Early and accurate diagnosis of brain tumors plays a vital role ... -
Muhasebe dersi alan öğrencilerin öğrenme stillerine göre öğrenim tercihlerinin belirlenmesine ilişkin bir araştırma: Gümüşhane Üniversitesi örneği
Akelma, Gülsüm (Gümüşhane Üniversitesi, 2019)Araştırmada, öğrencilerin kişisel özelliklerinin farklı olması dikkate alınarak, muhasebe öğrenim ve öğretiminde hızlı, kolay ve kalıcı bilgi için, öğrencilerin kişisel özellikleri ve yeteneklerine en uygun öğrenme stil ... -
DSCIMABNet: A novel multi-head attention depthwise separable CNN model for skin cancer detection
Reis, Hatice Catal; Turk, Veysel (Elsevier Ltd, March 2025)Skin cancer is a common type of cancer worldwide. Early diagnosis of skin cancer can reduce the risk of death by increasing treatment success. However, it is challenging for dermatologists or specialists because the symptoms ...