Deep Learning Based Focus Measurement Operator for 3D Imaging in Microscopic Systems
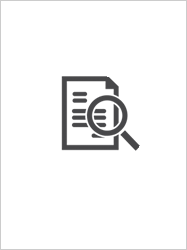
Access
info:eu-repo/semantics/restrictedAccessDate
2023Access
info:eu-repo/semantics/restrictedAccessMetadata
Show full item recordCitation
H. Doğan and R. Ö. Doğan, "Deep Learning Based Focus Measurement Operator for 3D Imaging in Microscopic Systems," 2023 31st Signal Processing and Communications Applications Conference (SIU), Istanbul, Turkiye, 2023, pp. 1-4, doi: 10.1109/SIU59756.2023.10224024.Abstract
Since 3D imaging in microscopic systems makes cell and tissue structures more prominent and visible, it provides higher performance in image processing applications such as automatic segmentation, recognition, classification, alignment and stitching. For this reason, 3D imaging in microscopic systems has been one of the most comprehensive and long-studied research areas in the literature. It seems that the most frequently preferred strategy for 3D imaging is shape from focus. Shape from focus is a strategy that uses a sequence of 2D images with the same perspective and different focused regions to create the 3D shape of the sample. Focus measurement operators are applied to extract the focus properties of the input images in shape from focus strategies. These operators have several limitations, such as more precise characterization of curves and edges in images. Researchers often use a pre- or post-processing technique to minimize these limitations, rather than proposing a new focus measurement operator. In this study, a deep learning based focus measurement operator, which does not require a pre- or post-processing technique, is developed to eliminate these limitations. The qualitative and quantitative results obtained in the study show that the proposed focus measurement operator is more effective in 3D imaging than other operators in the literature.