InSiNet: a deep convolutional approach to skin cancer detection and segmentation
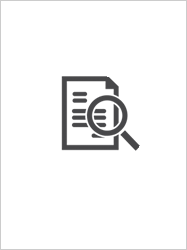
Access
info:eu-repo/semantics/restrictedAccessDate
2022Access
info:eu-repo/semantics/restrictedAccessMetadata
Show full item recordAbstract
Cancer is among the common causes of death around the world. Skin cancer is one of the most lethal types of cancer. Early diagnosis and treatment are vital in skin cancer. In addition to traditional methods, method such as deep learning is frequently used to diagnose and classify the disease. Expert experience plays a major role in diagnosing skin cancer. Therefore, for more reliable results in the diagnosis of skin lesions, deep learning algorithms can help in the correct diagnosis. In this study, we propose InSiNet, a deep learning-based convolutional neural network to detect benign and malignant lesions. The performance of the method is tested on International Skin Imaging Collaboration HAM10000 images (ISIC 2018), ISIC 2019, and ISIC 2020, under the same conditions. The computation time and accuracy comparison analysis was performed between the proposed algorithm and other machine learning techniques (GoogleNet, DenseNet-201, ResNet152V2, EfficientNetB0, RBF-support vector machine, logistic regression, and random forest). The results show that the developed InSiNet architecture outperforms the other methods achieving an accuracy of 94.59%, 91.89%, and 90.54% in ISIC 2018, 2019, and 2020 datasets, respectively. Since the deep learning algorithms eliminate the human factor during diagnosis, they can give reliable results in addition to traditional methods. Graphical abstract: [Figure not available: see fulltext.] © 2022, International Federation for Medical and Biological Engineering.
Volume
60Issue
3URI
https://link.springer.com/article/10.1007/s11517-021-02473-0https://hdl.handle.net/20.500.12440/5597