An automatic EEG-based sleep staging system with introducing NAoSP and NAoGP as new metrics for sleep staging systems
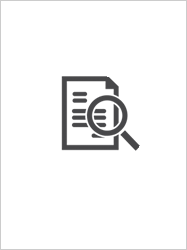
Access
info:eu-repo/semantics/closedAccessDate
2021Access
info:eu-repo/semantics/closedAccessMetadata
Show full item recordAbstract
Different biological signals are recorded in sleep labs during sleep for the diagnosis and treatment of human sleep problems. Classification of sleep stages with electroencephalography (EEG) is preferred to other biological signals due to its advantages such as providing clinical information, cost-effectiveness, comfort, and ease of use. The evaluation of EEG signals taken during sleep by clinicians is a tiring, time-consuming, and error-prone method. Therefore, it is clinically mandatory to determine sleep stages by using software-supported systems. Like all classification problems, the accuracy rate is used to compare the performance of studies in this domain, but this metric can be accurate when the number of observations is equal in classes. However, since there is not an equal number of observations in sleep stages, this metric is insufficient in the evaluation of such systems. For this purpose, in recent years, Cohen's kappa coefficient and even the sensitivity of NREM1 have been used for comparing the performance of these systems. Still, none of them examine the system from all dimensions. Therefore, in this study, two new metrics based on the polygon area metric, called the normalized area of sensitivity polygon and normalized area of the general polygon, are proposed for the performance evaluation of sleep staging systems. In addition, a new sleep staging system is introduced using the applications offered by the MATLAB program. The existing systems discussed in the literature were examined with the proposed metrics, and the best systems were compared with the proposed sleep staging system. According to the results, the proposed system excels in comparison with the most advanced machine learning methods. The single-channel method introduced based on the proposed metrics can be used for robust and reliable sleep stage classification from all dimensions required for real-time applications.