An EEG-based stereoscopic research of the PSD differences in pre and post 2D&3D movies watching
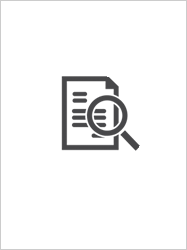
Access
info:eu-repo/semantics/closedAccessDate
2020Access
info:eu-repo/semantics/closedAccessMetadata
Show full item recordAbstract
Despite knowing the reality of three-dimensional (3D) technology in the form of eye fatigue, this technology continues to be retained by people (especially the young community). To check the influences of the human brain's power spectrum density (PSD) pre and post a 2D and 3D movie watching, a five-member test group was arranged. In this study, electroencephalogram (EEG) was used as a neuroimaging method. EEG recordings of five individuals were taken both before and after watching 2D and 3D movies. The main framework of this study was to analyze the effects of power spectrum density (PSD) of the human brain by testing all EEG frequency bands before and after 2D and 3D movie watching, also to determine effective brain lobes, and consequently, EEG channels. After 2D/3D EEG recording, this record was divided into three stages for analysis. These stages consisted of Relax, Watching, and Rest. This benchmarking analysis included 1) before and after watching the 2D movie (R2b and R2a), II) before and after watching the 3D movie (R3b and R3a), and III) after watching the 2D/3D movie (R2a and R3a). In the Relax and Rest stages, the 2D/3D EEG power differences in all channels of brain regions for the five EEG bands, including delta (delta), theta (theta), alpha (alpha), beta (beta), and gamma (gamma), were analyzed and compared. The PSD based on short-time Fourier transform (STFT) was used to select the dominant bands in this study. Feature extraction was performed on the preprocessed EEG signals using SIFT and discrete wavelet transform (DWT). In the 2D analysis, delta, theta, alpha, and beta acted as dominant bands, in 3D, beta, theta, and alpha were dominant bands, and in 2D/3D, delta and alpha were selected as meaningful and dominant bands. Partial least-squares regression (PLSR) and support vector machine (SVM) classification algorithms were considered in order to classify the obtained R2a and R3a EEG signals. After the dominant band selection, the correct choice of effective channel combinations in these bands resulted in the percentage of high success of classifiers for Stage III. Taking into account the optimum number of channels, the best percentage of classification accuracy for the average of PLSR and SVM classification results by considering the best channels of SVM and DWT feature extraction method is 91.83% and 80.73% respectively. (C) 2019 Elsevier Ltd. All rights reserved.