Safer-Driving: Application of Deep Transfer Learning to Build Intelligent Transportation Systems
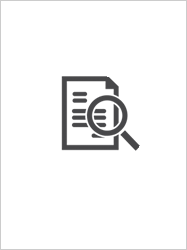
Erişim
info:eu-repo/semantics/closedAccessTarih
2021Erişim
info:eu-repo/semantics/closedAccessÜst veri
Tüm öğe kaydını gösterÖzet
Deep learning methods are widely used in the field of transportation as well as in many areas. These days, deep learning methods produce very successful results thanks to big data, where data collection and storage are much easier than in past years. However, it can be quite difficult to collect data or find the right data in some cases. On the other hand, even if the desired data is collected enough, training deep neural networks to learn from these data may not be comfortable in terms of hardware needed for ordinary users. In these cases, transfer learning can be an exit point, which is very popular in deep understanding, especially when the amount of data is limited. In this study, we have aimed to give comprehensive guidance on using a transfer learning strategy to create a detection model to establish a safer transportation system. More specifically, we have developed a classification model to alleviate accidents due to deer on the road. We have implemented VGG-16 convolutional neural network architecture as a part of the structured transfer learning framework. Computational results show that high accuracy performance can be achieved by implementing VGG-16 with the respectively small size of a dataset. © 2021, The Author(s), under exclusive license to Springer Nature Switzerland AG.