Advanced brain tumor analysis: a novel strategy for segmentation and classification using modern computational methods
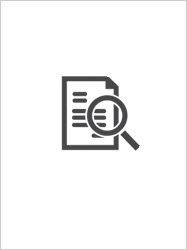
Erişim
info:eu-repo/semantics/openAccessTarih
February 2Erişim
info:eu-repo/semantics/openAccessÜst veri
Tüm öğe kaydını gösterKünye
Scopus EXPORT DATE: 12 March 2025 @ARTICLE{Reis20254697, url = {https://www.scopus.com/inward/record.uri?eid=2-s2.0-85213069607&doi=10.1007%2fs00521-024-10629-w&partnerID=40&md5=d55ff4425c3b2b1cd9ea62a2ba527d4c}, affiliations = {Department of Geomatics Engineering, Gumushane University, Gumushane, 29000, Turkey; Department of Computer Engineering, University of Harran, Sanliurfa, 63000, Turkey}, correspondence_address = {H.C. Reis; Department of Geomatics Engineering, Gumushane University, Gumushane, 29000, Turkey; email: hcatal@gumushane.edu.tr}, publisher = {Springer Science and Business Media Deutschland GmbH}, issn = {09410643}, language = {English}, abbrev_source_title = {Neural Comput. Appl.} }Özet
Despite their low incidence, brain tumors are one of the most invasive cancer types, constituting a significant burden of death and disease in all age groups. Early and accurate diagnosis of brain tumors plays a vital role in reducing mortality rates. The heterogeneous nature of brain tumors and the diversity of tumor lesions may make it difficult for radiologists to make the right decision in the manual diagnosis process. This study proposes the use of machine learning methods for the classification of brain tumors (pituitary, meningioma, and glioma) and the use of metaheuristic algorithms graph theory, and random walker algorithms in the segmentation of brain tumors. The classification performed with the proposed method obtained an overall accuracy rate of 98.33%. In addition, the classification accuracy of 99.50%, 99.50%, 98.67%, and 99.00% was achieved for no tumor, pituitary, meningioma, and glioma, respectively. Experiments in the segmentation process show that metaheuristic algorithms and max-flow graph cut approach produce successful results. © The Author(s), under exclusive licence to Springer-Verlag London Ltd., part of Springer Nature 2024.
Cilt
37Sayı
6Bağlantı
scopus.com/record/display.uri?eid=2-s2.0-85213069607&origin=SingleRecordEmailAlert&dgcid=raven_sc_affil_en_us_email&txGid=ffb1f5f24e766d17ac09febd1bda4adfhttps://link.springer.com/content/pdf/10.1007/s00521-024-10629-w.pdf?utm_source=scopus&getft_integrator=scopus
https://hdl.handle.net/20.500.12440/6469
Koleksiyonlar
İlgili Öğeler
Başlık, yazar, küratör ve konuya göre gösterilen ilgili öğeler.
-
Muhasebe dersi alan öğrencilerin öğrenme stillerine göre öğrenim tercihlerinin belirlenmesine ilişkin bir araştırma: Gümüşhane Üniversitesi örneği
Akelma, Gülsüm (Gümüşhane Üniversitesi, 2019)Araştırmada, öğrencilerin kişisel özelliklerinin farklı olması dikkate alınarak, muhasebe öğrenim ve öğretiminde hızlı, kolay ve kalıcı bilgi için, öğrencilerin kişisel özellikleri ve yeteneklerine en uygun öğrenme stil ... -
Integrated deep learning and ensemble learning model for deep feature-based wheat disease detection
Catal Reis, Hatice; Turk, Veysel (Elsevier Inc., 2024)Early detection of plant diseases is critical to prevent disease spread and assist farmers. Thanks to their high discrimination ability, Convolutional Neural Network (CNN)-based architectures can offer practical solutions ... -
DSCIMABNet: A novel multi-head attention depthwise separable CNN model for skin cancer detection
Reis, Hatice Catal; Turk, Veysel (Elsevier Ltd, March 2025)Skin cancer is a common type of cancer worldwide. Early diagnosis of skin cancer can reduce the risk of death by increasing treatment success. However, it is challenging for dermatologists or specialists because the symptoms ...