High Performance Automatic ROI Extraction from Hand Images with Convolutional Neural Networks
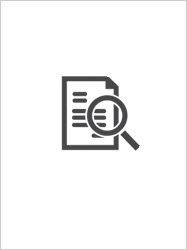
Erişim
info:eu-repo/semantics/openAccessTarih
21 SeptembErişim
info:eu-repo/semantics/openAccessÜst veri
Tüm öğe kaydını gösterKünye
Scopus EXPORT DATE: 06 March 2025 @CONFERENCE{Kosmaz Sunnetci2024, url = {https://www.scopus.com/inward/record.uri?eid=2-s2.0-85207886375&doi=10.1109%2fIDAP64064.2024.10710885&partnerID=40&md5=234ef9a4992cea992ad20525cd3b08f8}, affiliations = {Gumushane University, Department of Mathematical Engineering, Gümüşhane, Turkey; Gumushane University, Department of Software Engineering, Gümüşhane, Turkey; Karadeniz Technical University, Department of Software Engineering, Trabzon, Turkey; Karadeniz Technical University, Department of Computer Engineering, Trabzon, Turkey}, correspondence_address = {B. Kosmaz Sunnetci; Gumushane University, Department of Mathematical Engineering, Gümüşhane, Turkey; email: betul.kosmaz@gumushane.edu.tr}, publisher = {Institute of Electrical and Electronics Engineers Inc.}, isbn = {979-833153149-2}, language = {English}, abbrev_source_title = {Int. Artif. Intell. Data Process. Symp., IDAP} }Özet
Automatic ROI extraction from hand images is a critical step in palmprint recognition. Traditional methods involve steps such as identifying the region of the hand, finding the valleys between the fingers and marking the palm area. Some of these processes are performed manually and some are performed with image processing techniques. However, these methods are time-consuming, vulnerable to human error, and computationally redundant. In this study, a deep learning-based convolutional neural network is developed for high-performance automatic ROI extraction from hand images. The proposed method eliminates the hand segmentation process and provides a solution where the palm region can be automatically selected directly. This reduces processing time, minimizes human error and improves overall accuracy. © 2024 IEEE.
Bağlantı
https://www.scopus.com/record/display.uri?eid=2-s2.0-85207886375&origin=SingleRecordEmailAlert&dgcid=raven_sc_affil_en_us_email&txGid=af7c149f959d6a8a6accf18d8012c2cfhttps://hdl.handle.net/20.500.12440/6429