High-Accuracy Hand Segmentation for Palmprint Biometric Systems Improving Auto-Encoder Techniques
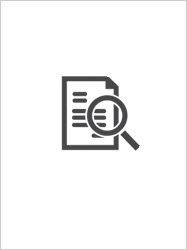
Erişim
info:eu-repo/semantics/openAccessTarih
6 DecemberErişim
info:eu-repo/semantics/openAccessÜst veri
Tüm öğe kaydını gösterKünye
Scopus EXPORT DATE: 06 March 2025 @CONFERENCE{Yalcin2024, url = {https://www.scopus.com/inward/record.uri?eid=2-s2.0-85218069585&doi=10.1109%2fISAS64331.2024.10845515&partnerID=40&md5=fb0998805815af00d69c598593ee5ba4}, affiliations = {Graduate Education Institute, Gumushane University, Department of Artificial Intelligence and Intelligent Systems, Gumushane, Türkiye; Trabzon University, Faculty of Computer and Information Sciences, Department of Artificial Intelligence Engineering, Trabzon, Türkiye; Karadeniz Technical University, Faculty of Engineering, Department of Software Engineering, Trabzon, Türkiye; Gumushane University, Faculty of Eng. and Natural Sciences, Department of Software Engineering, Gumushane, Türkiye}, correspondence_address = {K. Yalcin; Graduate Education Institute, Gumushane University, Department of Artificial Intelligence and Intelligent Systems, Gumushane, Türkiye; email: kadiryalcin@gumushane.edu.tr}, publisher = {Institute of Electrical and Electronics Engineers Inc.}, isbn = {979-833154010-4}, language = {English}, abbrev_source_title = {Int. Symp. Innov. Approaches Smart Technol., ISAS - Proc.} }Özet
Biometric technologies have been widely adopted in various commercial products, ranging from security systems to personal devices, due to their exceptional reliability and user-friendliness. Among these, palmprint biometrics has gained increasing attention for its advantages, such as ease of use, a larger surface area, and the ability to capture more features compared to other biometric methods. A typical palmprint biometric system involves five key stages: (a) Image Acquisition, (b) Hand Segmentation, (c) Pattern Generation, (d) Feature Extraction, and (e) Verification/Identification. The overall success of the system depends on the effectiveness of each stage, with hand segmentation being crucial for identifying the most relevant features for accurate verification. This study aims to achieve high-accuracy biometric recognition by segmenting hands from images captured in uncontrolled environments with complex backgrounds. Accuracy (ACC), Intersection over Union (IoU), F1-Score, and Dice Coefficient are the four critical metrics used to assess the performance of segmentation techniques. The findings demonstrate that the U-Net + ViT and MA-Net + ResNet34 techniques outperform traditional auto-encoder methods in both quantitative performance metrics and visual analysis, leading to significantly improved hand segmentation for biometric systems. © 2024 IEEE.
Bağlantı
https://www.scopus.com/record/display.uri?eid=2-s2.0-85218069585&origin=SingleRecordEmailAlert&dgcid=raven_sc_affil_en_us_email&txGid=32ee4cebd3fb952ad4b95a2801ca8585https://hdl.handle.net/20.500.12440/6423