In Different Scenarios MobileNet-V1 for Cross-view Gait Recognition
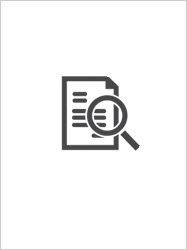
Erişim
info:eu-repo/semantics/openAccessTarih
4 SeptembeErişim
info:eu-repo/semantics/openAccessÜst veri
Tüm öğe kaydını gösterKünye
Scopus EXPORT DATE: 06 March 2025 @CONFERENCE{Yaprak2024, url = {https://www.scopus.com/inward/record.uri?eid=2-s2.0-85206477041&doi=10.1109%2fINISTA62901.2024.10683858&partnerID=40&md5=0e93de4c3a21a3af2042da34a801bbd0}, affiliations = {Gümüşhane University, Department of Software Engineering, Gümüşhane, Turkey; Karadeniz Technical University, Department of Software Engineering, Trabzon, Turkey}, correspondence_address = {B. Yaprak; Gümüşhane University, Department of Software Engineering, Gümüşhane, Turkey; email: busra.kucukugurlu@gumushane.edu.tr}, editor = {Badica C. and Ivanovic M. and Koprinkova-Hristova P. and Leon F. and Manolopoulos Y. and Yildirim T. and Ucar A.}, publisher = {Institute of Electrical and Electronics Engineers Inc.}, isbn = {979-835036813-0}, language = {English}, abbrev_source_title = {Int. Conf. INnov. Intell. Syst. Appl., INISTA} }Özet
Gait recognition aims to identify people from a distance by analyzing their walking style. Nevertheless, the efficacy of recognition drops significantly under cross-view and, appearance-based variations such as carrying and clothing. In this study, the performance of the MobileNet-V1 deep network is evaluated in various scenarios to address the cross-view gait recognition problem. In the first scenario, the fine-tuned MobileNet-V1 is evaluated on Gait Energy Images (GEI) as input data, while in the second scenario, the fine-tuned MobileNet-V1 is assessed with Optical Flows and masked RGB frames input data. In the last scenario, the first two scenarios are combined over a single fused deep network based on finetuned MobileNet-V1, and a single recognition process is performed using two different fused features data; GEI features with Optical Flow features, and GEI features with masked RGB frame features. In the evaluation process, a comprehensive data set for the cross-view gait recognition problem, CASIA-B is used for the experiments. The obtained results demonstrate that in the last scenario, the contribution of masked RGB frame features to the recognition rate of GEI is more significant. © 2024 IEEE.
Bağlantı
https://www.scopus.com/record/display.uri?eid=2-s2.0-85206477041&origin=SingleRecordEmailAlert&dgcid=raven_sc_affil_en_us_email&txGid=e0b169034a7d168bbbadfd222c4d8ae7https://hdl.handle.net/20.500.12440/6410